Shedding Light on the Black Box of Sentiment Analysis
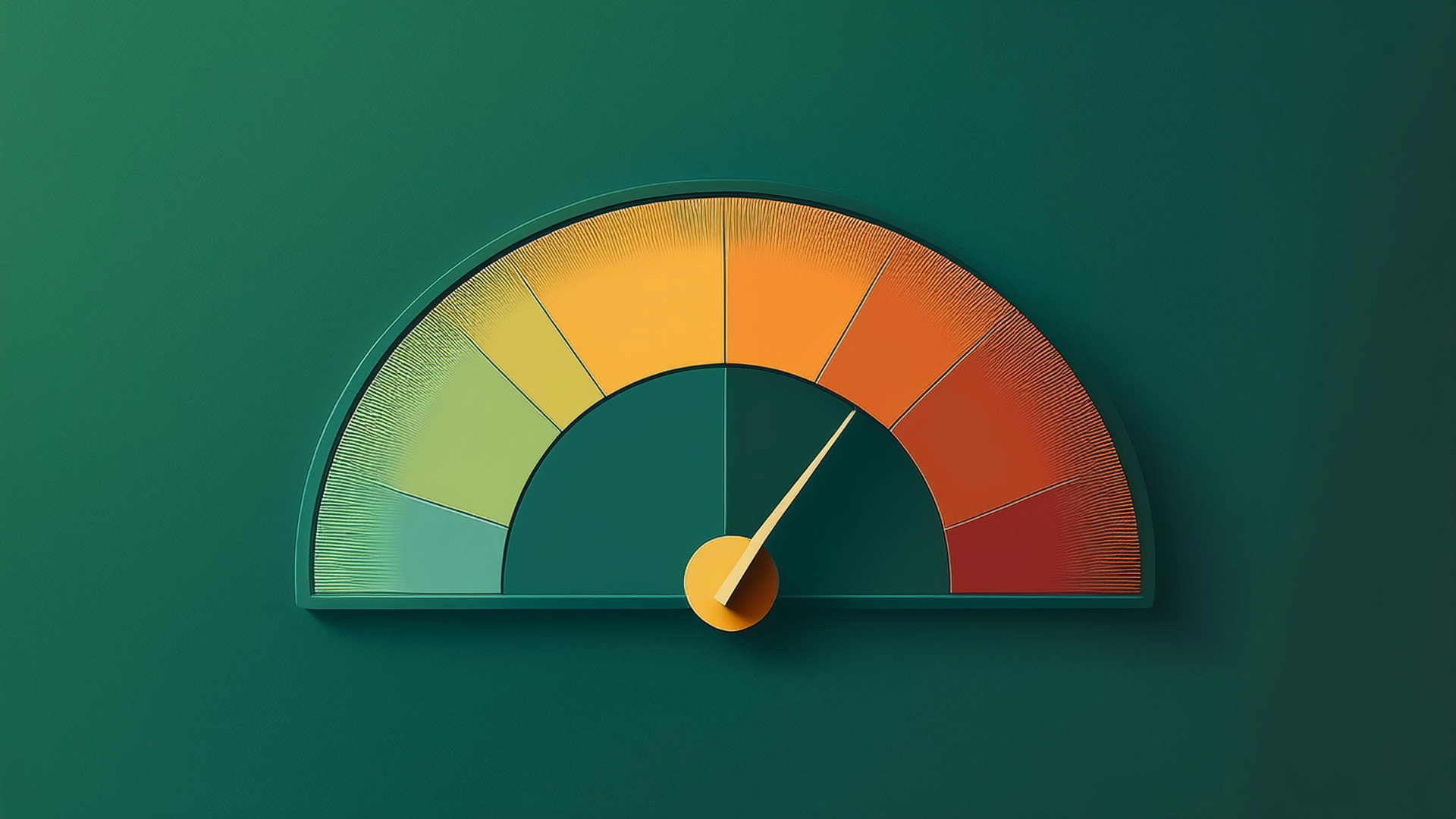
A stakeholder crisis is coming for every business; 95 percent of executives agree, but preparedness takes more than awareness—it requires actionable insights and strategic planning. As communicators, we play a pivotal role in understanding our stakeholders and navigating future challenges effectively by using sentiment analysis. Whether you aim to gauge customer satisfaction, monitor brand reputation, or tailor marketing strategies, sentiment offers unique insights into the emotions beneath the words. However, many sentiment analysis tools fall short, misunderstanding the nuances of language and failing to grasp broader context.
Traditional approaches to sentiment analysis miss the mark in two critical ways: they rely on lexicon-based methods that often misinterpret context and sarcasm, and they provide a generic analysis of the entire content piece without considering the writer's perspective towards individual entities such as companies, executives, or topics. As a result, the output from these models can be inaccurate, leading to misguided decisions and a failure to understand your stakeholders.
At Penta, we combine our proprietary topic classification and sentiment engines with leading large language models (LLMs) to provide context-aware insights that truly reflect stakeholder perception. Here are five steps we take to achieve increased accuracy over conventional approaches:
1. Understand the content
To deliver maximum value, we calculate sentiment for both specific entities mentioned within a content piece and the piece as a whole. This approach provides a comprehensive overview, offering both a high-level perspective and detailed insights into how your topics are perceived. Our methodology highlights where communication efforts need to be placed by enhancing your understanding of news articles, social media posts, earnings reports, transcripts and more.
Tracking organizations, individuals, and topics is the first step to understand content. Our classification system accurately tags mentions of entities within content pieces, enabling us to easily identify references to your organization, your competitors, and relevant issue areas. This deep understanding of who and what is mentioned provides the necessary context for meaningful analysis.
2. Leverage industry expertise
Our unique ability to synthesize in-house industry expertise and combine it with the data crunching capabilities of LLMs allows us to deliver consistent and accurate sentiment calculations as if done by an analyst.
Out of the box, LLMs produce responses based on a general set of training data, but their true power emerges when they are guided with precision. This is done through the thoughtful implementation of system-level prompts. System-level prompts are predefined instructions that govern the behavior of the model. This technique allows us to define the role of the AI, guide its responses, and maintain consistency throughout interactions, which is crucial for applications that require stable and predictable behavior.
Our system prompt has been refined using insights gained from 15 years of assessing business context sentiment as conveyed in communicated content. It evaluates expressed views using a methodology developed by our industry experts through content assessment and review to clearly articulate scoring criteria. This level of granularity allows us to understand why our sentiment engine produces a given score, demystifying the “black box” concerns found elsewhere.
3. Simplify the output
We score sentiment on a scale from -100 to +100, providing an intuitive and granular approach compared to simple positive, neutral, and negative labels. This enables tracking of small sentiment changes over time, leading to powerful derivative solutions like our award winning Reputation Intelligence platform.
4. Monitor output quality
AI is not a set-it-and-forget-it tool. Updates to foundation models can alter performance, with the potential to negatively affect sentiment scores. Regular review of sentiment engine outputs is essential to maintain accuracy. We employ automated checks to assess model drift in real-time, enabling rapid issue resolution.
In addition to automated tests, analysts constantly review sentiment scores to ensure they accurately reflect the media landscape. All manual changes are cataloged, allowing us to review human feedback and adjust our systems accordingly. This feedback loop sets us apart by combining advanced automation with expert human oversight to deliver reliable and impactful results.
5. Interpret Insights
While you can view and interact with much of our data via the Penta Live platform, many of our solutions are managed by internal teams, customized to the exact specifications of the engagement. This bespoke approach ensures that a dedicated Penta industry expert is with you every step of the way. They provide the human benefit of interpreting and contextualizing the data, allowing you to focus on leveraging actionable insights rather than learning a new user interface.
When done right, sentiment analysis is a great asset to the modern communicator, allowing you to better understand your stakeholders and get ahead of narratives negatively impacting your brand. Our approach to sentiment analysis provides an accurate picture of individual entities mentioned within content and offers a comprehensive understanding of your reputation, and that of your peers.